Project Management Methodologies for Big Data Analytics
Published on 28 August 15
0
1
Many companies today are in the very early stages of adopting Big Data analytics. They are likely to go down a road ahead filled with experimentation and discovery. From many decades of IT history it is known that a significant number of large projects or new initiatives just end up in failure, or do not deliver all the results they promised. Business analytics is not the same as IT, of course, but there is a significant element of IT work in analytics. In addition, analytics usually involves multidisciplinary teams working with a lot of uncertainty.
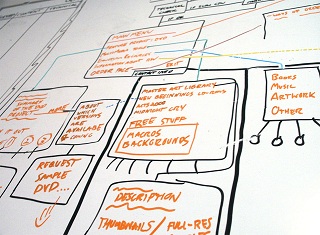
Appropriate management methodologies. What are the lessons from the past decades of IT experience that can be learned from in terms of managing analytics work better? One approach is to imbibe the perspectives of the CMMI along with Agile methods. The CMMI (or Capability Maturity Model Integration) is all about maturing an organization's system of developing software so that it’s outcome becomes more repeatable, predictable, and constantly improving in terms of assuring a quality output. The Agile methods address the project execution level, and aim at reducing overall project delivery risk by breaking down a project into nibbles at backlogged requirements, while allowing for the introduction of new or changing requirements in a more controlled way. In addition, Agile methods advocate a high degree of collaborative and coordinated working between empowered individuals in various project lifecycle roles, and this suits the circumstances of projects that involve a lot of uncertainty.
Unavailability of past metrics. Common causes of IT project failure have to do with unclear requirements, estimation of effort and cost based on poor assumptions or too many unknowns, poor management of stakeholder expectation, unrealistic goals, and so on. It is rarely because of a lack of understanding of technology and working processes. Big Data analytics projects, however, may be starting off with no in-house precedence to provide reference metrics on effort, productivity, and resourcing difficulties. And although there is a lot of high level information about various tools and techniques available, the devil is in the details, and there may not be enough specialists at the starting line to help uncover it all at the right time.
The value of a PoC. Big Data projects typically require a lot of effort to be spent on data extraction, cleansing and integration. At the same time, data volumes involved are, by definition, inherently large, and therefore as in the case of IT, it may be best to define a Proof of Concept project as a first step, one which is clearly communicated to all stakeholders as starting with only best guess estimates of time, cost and the probability of success. A proof of concept project executed over a few agile iterations will help uncover many unknowns, not only in terms of project outcomes, but also in terms of a clearer understanding of what processes work better, how much effort they need, and so on.
Achieving repeatability. Once the PoC project is complete, it would be worth spending some time reflecting on the course of the project and documenting the first set of learnings that will form the foundation for future repeatability. Some of the questions to ask are:
- What should be made standard in terms of work processes, environment preparation and technology usage?
- What’s the best approach to data sourcing and cleansing?
- What were the timelines and effort associated with each work task?
- Will these likely be reduced in future projects?
- Can new guidelines be prepared in any area?
- Can training material be prepared?
- What’s the QA strategy that works?
- Is there any scope for automation of any tasks?
Agile analytics. Once data is available, and can be used for statistical analysis and modelling the agile approach involving either full-fledged data scientists, or a multidisciplinary team of statistical analysts and business domain experts supported by an IT developer would again be useful, with successive iterations of work leading to discovery of insights and a refined model. Once the model is validated it can then be introduced to the business with appropriate training so that they can continue to use it on their own moving forward.
Management communication. Throughout the whole cycle, it is important to manage expectations through timely and factual communication. When the business has invested in an initiative that involves uncertainty to begin with, providing them with status and progress updates proactively and regularly improves the chances of their buy-in of the concept and results at a later stage. In addition it helps manage their expectations in the event that estimated schedules are overrun, or budgets don’t return business benefits as anticipated. Early failures and early successes communicated well could both lead to a level of understanding that leads to continued funding, and ultimately the desired bigger successes.
This blog is listed under
Development & Implementations
, Data & Information Management
, Project & Service Management
and IT Compliance & Audit
Community
Post a Comment
You may also be interested in
Share your perspective

Share your achievement or new finding or bring a new tech idea to life. Your IT community is waiting!